Predictive analytics and AI have not just changed the game; they've revolutionized it. This dynamic duo has transformed how companies handle staffing needs and manage personnel resources. By grasping the significance of predictive analytics and its symbiotic relationship with AI, businesses can gain a strategic advantage in the competitive market and achieve remarkable organizational success.
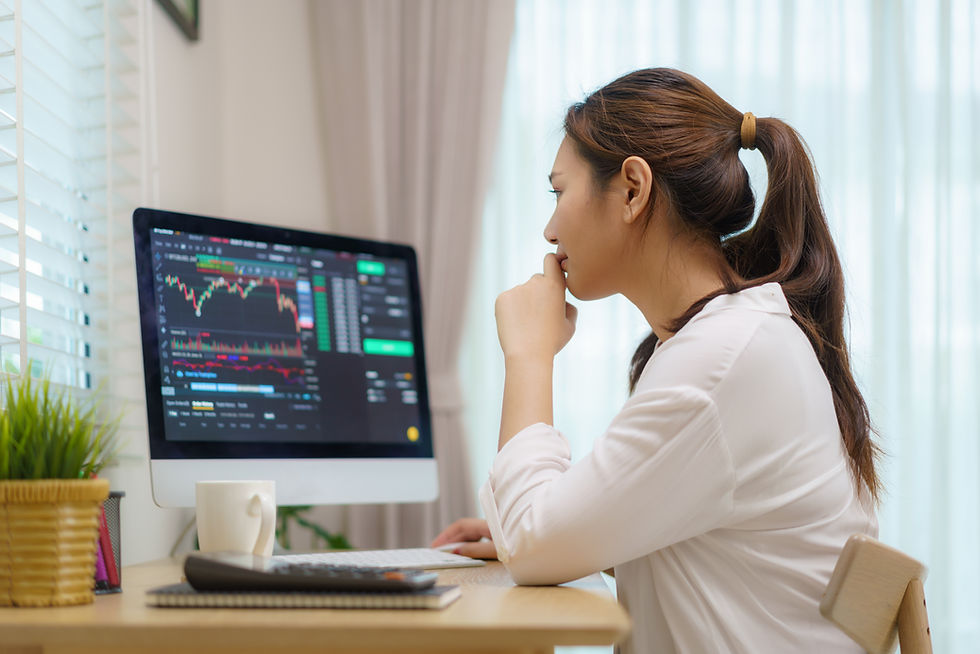
The Fundamentals of Predictive Analytics in Workforce Management
Predictive analytics is a data analytics field in which statistical algorithms, machine learning, and historical data are utilized to create models for predicting future outcomes. This technique is widely used in various fields, including workforce management. In workforce management, predictive analytics involves analyzing patterns, trends, and relationships across various employee-related metrics, such as performance, attrition rates, hiring cycles, and other relevant factors. By leveraging these insights, companies can predict future staffing requirements and proactively plan their workforce needs.
The predictive analytics approach is not just about reacting to the present; it's about preparing for the future. By anticipating future needs, companies can optimize workforce management strategies, reduce costs, and enhance overall operational efficiency. Predictive analytics is a powerful tool that enables companies to detect potential issues before they become significant problems. It empowers them to take proactive corrective measures and prevent any adverse impact on their business. Predictive analytics can offer an edge to organizations in this fast-changing business environment by keeping them ahead of the curve.
The Pivotal Role of AI
Creating efficient and accurate predictive models is a cornerstone of business success. However, this task is daunting due to the vast amounts of data that must be analyzed. This is where AI steps in, pivotal in powering these predictive models. By sifting through massive datasets, AI can learn from trends and anomalies, constantly refining its predictions to enhance accuracy.
The importance of AI in this domain cannot be overstated. It empowers predictive models to adapt and improve, enabling businesses to make reliable forecasts on staffing needs, even amidst the ever-changing business landscapes. By leveraging AI, companies can optimize their operations and ensure they have the right people in the right place at the right time. This can increase productivity, improve customer service, and improve financial results.
Driving Efficient Staffing with Predictive Analytics
Anticipating staffing needs is essential to maintaining operational efficiency and meeting business objectives. Predictive analytics fueled by AI is transforming this process.
Forecasting Demand: By analyzing past business performance and market trends, companies can predict and prepare for periods of high demand by proactively staffing their workforce to align with expected business volumes.
Identifying Skill Gaps: Organizations can use AI-driven analytics to forecast future skill requirements and address gaps through targeted recruitment or training programs before they impact business operations.
Reducing Attrition: Predictive models can help companies identify factors that lead to high turnover rates. This knowledge enables them to implement retention strategies tailored to keep their workforce engaged and reduce costly turnover.
Optimizing Talent Acquisition: Companies can enhance their employer brand and tap into talent pools by strategizing recruitment efforts to predict challenging roles.
Challenges and Considerations
The utilization of predictive analytics has gained immense popularity in managing workforces. The ability to anticipate future workforce trends and identify potential challenges before they occur can significantly improve business outcomes. However, implementing predictive analytics has its challenges.
Ensuring data quality and integrity is critical to produce accurate predictive models. It's important to understand that the accuracy of the predictions directly depends on the quality of the data fed into them. As such, organizations must prioritize collecting and analyzing correct data to produce trustworthy and reliable results. Additionally, ethical considerations around privacy and bias must be taken into account. Predictive models can inadvertently disadvantage certain groups of employees, which can have legal and reputational repercussions.
Despite the power of predictive analytics, the human element remains crucial. While AI-driven predictions can provide valuable insights, they must be interpreted and implemented by knowledgeable professionals who understand the nuances of their organization's culture and strategic goals. This human touch makes the difference in the success of AI-driven strategies. Only with the right expertise and experience can organizations leverage the full potential of predictive analytics to achieve their workforce management goals.
Success Stories: AI in Action
Several prominent organizations have recently harnessed the power of AI-driven predictive analytics to enhance their workforce management strategies. A leading global retail chain is a prime example. By utilizing AI-driven predictive models, the company accurately predicted the demand for its products and services during peak hours and adjusted its staffing levels accordingly. This strategic move enhanced customer satisfaction and led to a significant increase in sales, showcasing the tangible benefits of AI in workforce management.
Similarly, another technology company utilized AI to predict the most in-demand skills for the future. The company implemented AI-powered predictive models to analyze data from various sources, such as job postings, industry reports, and employee surveys. Based on this analysis, the company was able to identify the skills that would be most relevant and sought-after in the future. This helped the company to align its employee development programs and recruitment strategies accordingly, creating a future-ready workforce.
The Future Is Now
The merging of predictive analytics and AI is a significant shift in the approach towards workforce management and is not just a passing trend. With the constantly evolving technology, these tools can offer even more profound insights and accurate predictions. Integrating these technologies empowers businesses to move towards data-driven forecasting in their strategic planning, providing them with a competitive edge.
AI's role in anticipating staffing needs through predictive analytics is transformational. It enables businesses to shift from reactive personnel management to a proactive strategic stance that anticipates changes, prepares for the future, and navigates the complexities of today's dynamic business environment. The integration of AI in workforce management is expected to deepen, providing new opportunities for optimization and innovation.
However, while navigating these waters, businesses must balance technological possibilities and ethical imperatives of fairness, privacy, and transparency. Organizations that successfully achieve this balance will optimize their workforce and foster a more engaged, productive, and resilient workforce ready to face the future's challenges head-on. This commitment to ethical considerations ensures the responsible use of AI in workforce management.
The transformative power of AI in predictive analytics for workforce management is a remarkable testament to how technology can be harnessed to enhance human labor, not just replace it. As we continue to explore this frontier, the potential for creating more efficient, responsive, and adaptable organizations is virtually limitless. With AI and predictive analytics integration, businesses can be prepared for future changes, plan strategically, and gain a competitive edge in the market.
Comments