Navigating Ambiguity: Lessons Learned from Messy Data
- Pang the Analyst
- Feb 8, 2024
- 5 min read

Introduction
In today's world of big data, we often hear about the precision of machine learning models or the accuracy of advanced analytics. However, anyone who has worked with real-world data knows it is rarely clean or straightforward. In fact, ambiguity and messiness are the norms in data science rather than the exceptions. In this blog, we will delve into the challenges posed by ambiguous and messy data and explore the lessons learned through a case study. We will discuss strategies to manage these challenges and suggest best practices for extracting valuable insights from less-than-perfect datasets.
Embracing Imperfection: The Reality of Real-World Data
In the modern world, data has become the foundation of successful businesses. It is undeniable how crucial data is in organizations' strategic decision-making process. However, not all data is created equal. Analysts and data scientists often come across data that requires completion, consistency, or clarification due to various factors such as human error, limitations in the data collection process, or changes in data formats. These imperfections can pose significant challenges and hinder data analysis's smooth functioning and accuracy.
Understanding the Scope of Ambiguity in Data
Data ambiguity can occur when some analysts need clarification formation in multiple ways, leading to confusion and uncertainty. One typical example is customer feedback forms that contain a free text field for "service rating." Responses like "good," "fast," or "friendly" may seem optimistic, but they don't provide a clear indication of the customer's experience in a statistical form. This type of data can be challenging to analyze and draw insights from.
Messy data, on the other hand, often contains missing values, duplicates, or errors that can create confusion and hinder analysis. Imagine a sales database where some transactions lack product codes, or the same customer appears multiple times with slight variations in the name. Such data can be challenging to clean and standardize, leading to inconsistencies and inaccuracies in the analysis. Being able to identify and address these issues is essential to ensure that the data is trustable and helpful in making informed decisions.
Case Study: Messy Data in Retail Analytics
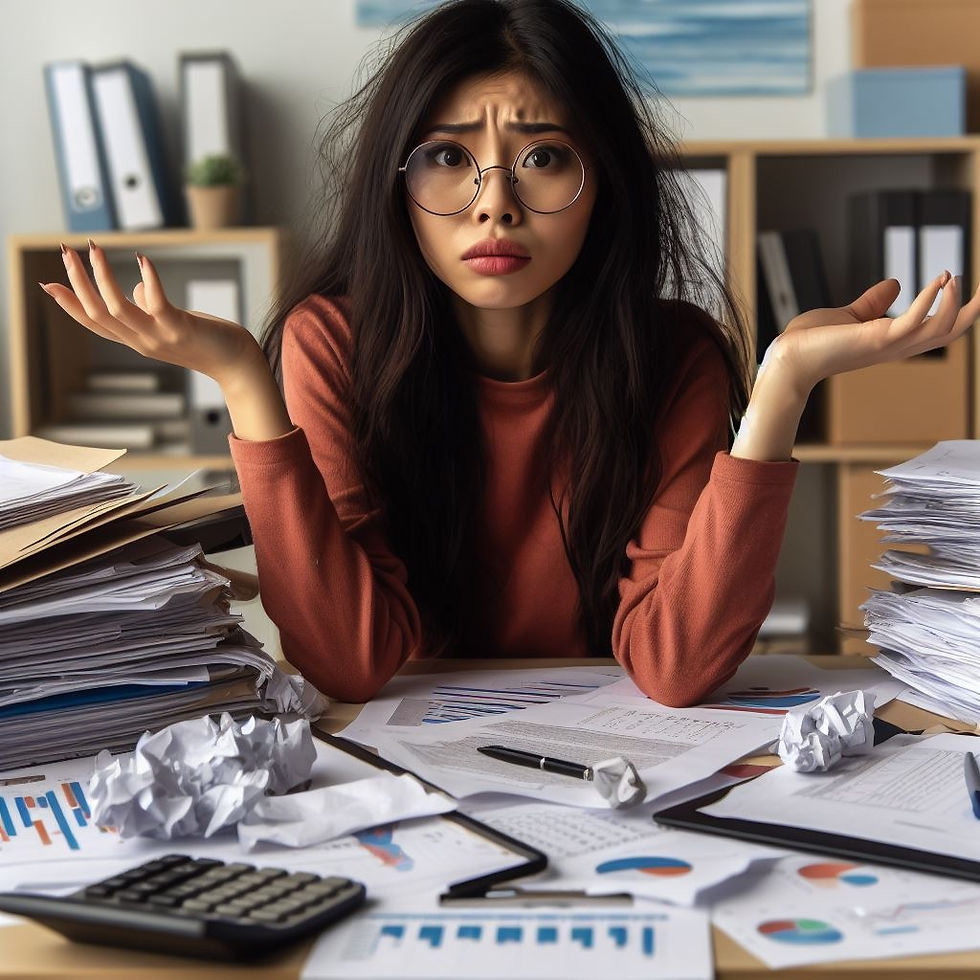
Consider the following scenario in the retail industry. A retailer of moderate size had a strategy to utilize their customer transaction data to create personalized marketing campaigns. However, they faced a difficult challenge. Their data could have been more accurate, including incomplete transaction records, inconsistent customer information, and mixed formatting resulting from merging legacy systems.
The Strategy for Tackling Messy Data
The company utilized a systematic approach to address the challenges posed by its disorganized data. The first step involved data profiling, thoroughly analyzing the data's quality and structure. This involved scrutinizing everything from missing values and outliers to patterns of inconsistency. With the insights gained from this assessment, the team could prioritize the issues based on their impact on analysis. By taking these measures, the company could better manage its data and improve the accuracy and reliability of its analyses.
Data Cleaning and Preparation
The team managing the retailer's data began a challenging data-cleaning task. To ensure the data's accuracy and completeness, they employed several techniques, including data imputation, standardization, and error correction.
In data imputation, they used various statistical methods or business logic to fill in the gaps for missing data fields. For instance, if a particular record had a missing country field, they could deduce the country using the postal code. This helped ensure that the data was complete and consistent.
They also used standardization techniques to unify various representations of the same name. This helped to reduce duplicates and inconsistencies in the data. By standardizing the data entries, they could quickly identify and merge duplicate records, which helped streamline their data management processes.
Finally, they employed error correction techniques to flag outliers and potential errors for manual review. Sometimes, they had to revert to the source documents or systems to validate the information. This helped ensure the data was accurate and reliable, critical for the retailer's operations and decision-making processes.
Data Enrichment and Transformation
The team took additional measures to make the data more useful by incorporating external data sources. One method they employed was utilizing demographic data linked to specific postal codes to gain insights into customer segments. In addition to this, they also made structural changes to the data to ensure it was compatible with their analytical models. This ensured that all the information was aligned with their intended marketing strategies, allowing for a more effective decision-making process.
Building Analytics on a Solid Foundation
By refining the quality of their data, the team could construct a comprehensive analytics framework equipped with advanced capabilities that were crucial for their business. They utilized innovative clustering algorithms to partition customers into distinct groups based on their purchasing tendencies and employed cutting-edge predictive models to predict future buying behaviors. Armed with these valuable insights, the team was able to execute highly effective and targeted marketing campaigns, resulting in a significant boost in sales and customer satisfaction.
Iterative Improvement: The Virtue of Repetition
The retailer recognized the importance of maintaining accurate and clean data and acknowledged that it was not a one-time process but an ongoing effort. They regularly revisited their data-cleaning strategies, refining their models and customer segmentation. They observed that certain data transformations improved the performance of their models, leading to a virtuous cycle of data quality improvement that informed their cleaning process. Through this iterative approach, the retailer was able to optimize their data quality and enhance their business operations.
Culture Shift: From Clean Slates to Cleaning Brooms
During the training session, the team learned a crucial lesson about the cultural shift in the organization. The session emphasized the importance of moving away from the expectation of perfect data and accepting the reality of data messiness. As a result, the team was able to develop processes that incorporated data cleanup as a critical component of the data management cycle. This shift in mindset allowed the team to work more efficiently and effectively with data, ultimately leading to improved outcomes.
Tools and Technologies: Choosing the Right Equipment
The retailer effectively utilized open-source and commercial tools to analyze and manage their data during their journey. To automate the process of cleaning their data, they used scripting languages like Python and R. Additionally, they employed Apache Spark, a mighty big data processing engine, to handle large datasets efficiently. They also utilized data visualization tools like Tableau to extract meaningful insights from the processed data. This helped them identify patterns or anomalies in the raw data tables that might have otherwise gone unnoticed, thus enabling them to make more informed decisions.
Lessons Learned
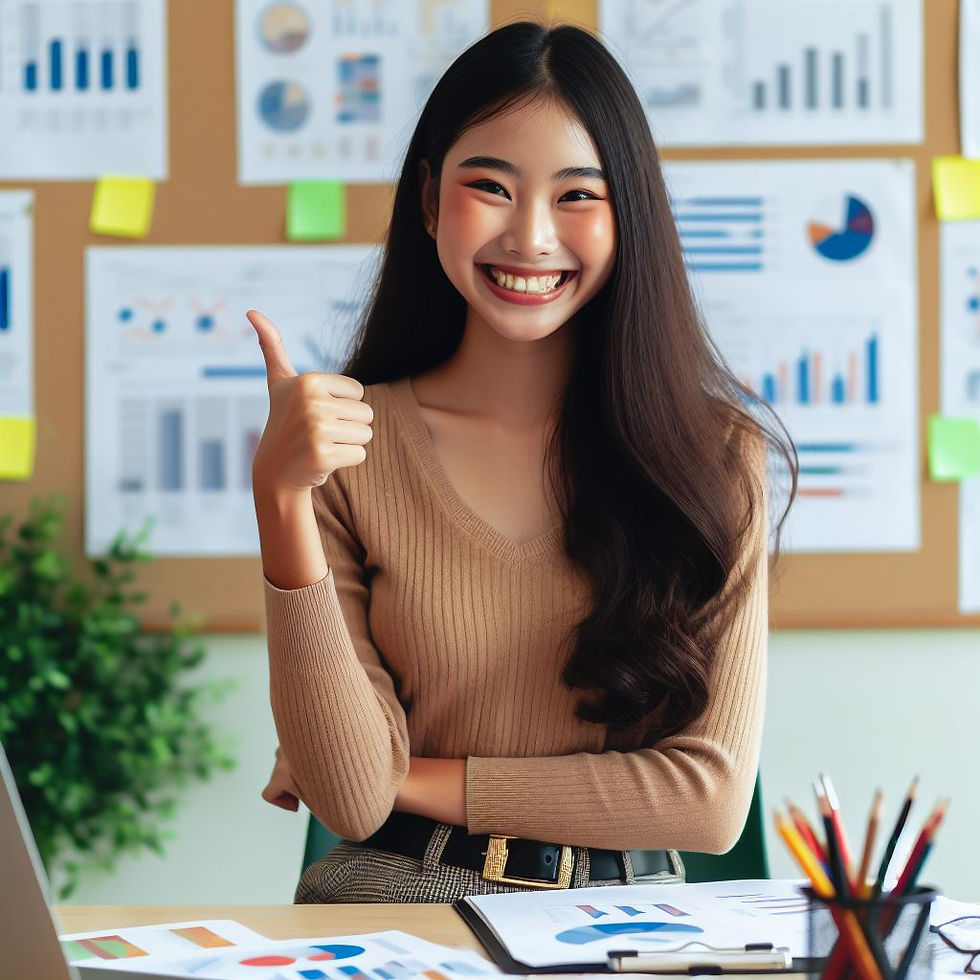
This case study has highlighted some critical lessons that can be helpful in data management and cleaning. One of the key takeaways is that early and continuous data quality assessment can prevent significant future issues. Additionally, investing in training to master data cleaning and management tools can pay off significantly. It is also essential to integrate data cleaning as a part of the data lifecycle instead of treating it as a one-off project. Finally, managing stakeholder expectations around data quality and timelines is crucial for delivering usable insights without overselling the certainty of those insights.
Conclusion
In today's data-driven world, navigating the ambiguity inherent in messy data is essential for decision-making and business success. Achieving this requires combining technical skills, strategic thinking, and a realistic approach to data quality. Our retail case study demonstrates how treating data cleanup as a continual process, selecting appropriate tools, and fostering a data-centric culture can transform messy data from a liability into an asset. By embracing the imperfection of real-world data, businesses can uncover valuable insights that inform decision-making in powerful, albeit sometimes unexpected, ways. In conclusion, it is imperative for organizations to adopt a pragmatic approach to data quality and appreciate that messy data can be a valuable resource when appropriately managed.
Comments